Snowflake 2023-03-07 Morgan Stanley Technology, Media & Telecom Conference
发布时间 2023-05-01 04:13:07 来源
中英文字稿 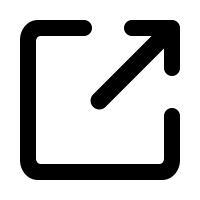
All right.
Thank you everyone for joining us. My name is Keith Weiss. I run the US software research group here at Morgan Stanley. And very pleased to have with us Mike Scarpelli, CFO and Christian Kleinman, SVP product from Snowflake.
好的。感谢大家参加本次活动。我是Keith Weiss,目前在摩根士丹利担任美国软件研究小组负责人。同时,我们很荣幸地邀请到Snowflake的CFO Mike Scarpelli和SVP产品Christian Kleinman参加本次活动。
So thank you gentlemen for joining us.
Thanks for having us.
Thank you.
感谢各位先生能够加入我们。
谢谢邀请。
谢谢。
Before we get started, a brief disclosure for important disclosures, please see the Morgan Stanley Research School of your website at www.morganfamily.com backslash research disclosures. If you have any questions, please reach out to your Morgan Stanley sales representative.
在开始之前,需要做一些简要的声明。如需重要的声明,请访问摩根士丹利的研究网站www.morganfamily.com/research disclosures。如果您有任何问题,请与您的摩根士丹利销售代表联系。
Excellent. So that out of the way.
So actually I want to get started in the presentation with you Christian and talking about the market opportunity ahead of Snowflake.
非常好,那就这样先说过了。现在,我想与你克里斯蒂安一起开始演示,谈谈Snowflake未来的市场机会。
I think one of the most impressive parts of the story is how that opportunity is evolved over the past couple of years.
I remember at the IPO, we were talking about roughly an 80-81 billion dollar market opportunity, but you guys have developed into sort of the adjacencies around your core business.
And now we're talking about $248 billion in market opportunity.
Can you walk us through the steps of how we got there, how we expanded out that opportunity?
我认为这个故事最令人印象深刻的部分之一就是在过去几年中商机的发展。我记得在首次公开募股时,我们谈论了大约800-810亿美元的市场机会,但是你们已经发展出了核心业务周围的相关领域。现在,我们正在谈论价值2480亿美元的市场机会。能否向我们解释一下,我们是如何通过扩展机会获得的,其中经历了哪些步骤?
Yeah, so the early days of Snowflake were all about helping organizations break down silos and consolidate their data.
If you look at pretty much every large organization they have a little bit of vertical and these are all these different data with technologies and it's hard for them to think across. So our thesis was let's help organizations combine the data and be able to think throughout the business.
嗯,Snowflake 的早期日子都是在帮助组织打破信息孤岛,整合他们的数据。如果你看看大多数大型组织,它们都有一些垂直领域,这些领域使用不同的数据技术,难以进行跨领域的考虑。因此,我们的理论是要帮助组织合并数据,并能够在业务方面进行全面的思考。
And then what we saw is even when customers have been able to consolidate data, they keep finding reasons to start to copy bits and pieces of their own data into different systems.
Oh, I have an application that does some AI, so I copy the data. I have an application that does some graph processing I copy the data.
And our whole thesis is instead of re-silowing the data, how do we help customers bring that application, those business logic, into Snowflake, and that's when you hear us talking about Snowflake as an application platform, which dramatically changes the scope of what we do.
接着,我们发现即使客户已经成功地整合了数据,他们仍然会找到各种理由将自己的一些数据复制到不同的系统中。例如,我有一个应用程序可以做一些人工智能任务,所以我会复制数据。我有一个应用程序可以进行一些图形处理,我也会复制数据。
我们的整个理论是,我们不是把数据重新进行隔离,而是如何帮助客户将那些应用程序和业务逻辑,带入到Snowflake中。这就是我们谈到Snowflake作为一个应用平台的时候,它极大地改变了我们的工作范围。
And intersecting this type of business logic on Snowflake, we're also very focused on helping organizations collaborate with data.
That's where data sharing technology fits in, that's where data, clean room technology fits in. And the intersection of all of this as we have to do for us, keeps getting larger and larger.
在Snowflake上交叉应用这种业务逻辑的同时,我们也非常专注于帮助组织协作使用数据。这就是数据共享技术和清洁室技术的应用场景所在。而所有这些的交叉应用对我们来说,越来越重要。
Got it. I think the data sharing element is probably one part of the Snowflake story.
I think people still under appreciate.
The way I think about it, in sort of the old data warehousing technologies, pricing was based on capacity, like how big is your data warehouse.
In the Snowflake model, 90% is compute.
How many questions are you asking of the data?
And in every company that I talk to, one of the primary reasons for moving into a cloud-based data warehouse, it's to enable more sharing and enable more people to ask questions of that data.
So I think there's an inherent expansion of the market opportunity that comes just from moving to the cloud, and just from getting that data sharing.
明白了。我认为数据共享是Snowflake故事的一部分,这可能是人们仍然没能充分体会到的一部分。在我看来,以前的数据仓库技术的定价是基于容量的,比如你的数据仓库有多大。在Snowflake的模式中,有90%是计算能力。你对数据提出了多少问题?在我跟每个公司的交流中,我发现转向基于云的数据仓库的主要原因之一是为了更多地共享和让更多的人问那些数据问题。因此,我认为这种转向云的运动本身就存在着市场机会的潜在扩大,只要进行更多的数据共享即可。
And you kind of kind of track the edge.
Can you explain to us kind of what edges are and sort of how you see that developing within the base?
“Kind of” 代表有点、有些,意思是你有点追踪边缘。你能解释一下什么是边缘,以及你如何看待它在基础的发展中起到的作用吗?
So we think of sharing or enabling sharing of data, both within an organization, but also across organizations.
And both are obviously very important and meaningful opportunity for us.
The way we think of sharing relationships is what we call an edge, which is the connection of two organizations or two parts of an organization, where they have activity one querying data from the other.
因此,我们考虑分享或促进数据的共享,既可以在一个组织内部,也可以跨组织进行。而且这两者对我们来说都非常重要和有意义的机会。我们考虑共享关系的方式是我们所说的“边缘”,即两个组织或组织的两个部分连接处,在这里它们可以通过查询其他组织的数据进行活动。
And that's what we call an active edge. So if I share there with you heat, we have an edge.
But the number that you hear or the metric that you hear us talk about is what we call stable edges, which are edges that have a minimum threshold of activity over a minimum amount of time, which tends to suggest that this is not a one-off conversation mic and I did, but it's a persisted ongoing relationship.
I don't know if you want to add anything?
这就是我们所说的活性边缘。如果我和你分享了动态信息,我们就会有一个边缘。但是,我们所提及的数字或指标是稳定边缘,它们具有在一段最短时间内的最小活动阈值。这意味着这不是一次性的对话,而是一种持续的关系。你想补充些什么吗?
No, that data sharing really creates a stickiness in terms of we're actually seeing RFPs out there from some of our customers are actually asking their vendors questions, are you a snowflake customer because they want to do data sharing.
不,数据共享真的会带来粘性,因为我们实际上看到一些客户发布RFP,询问他们的供应商是否是雪花客户,因为他们想要进行数据共享。
So beyond just stickiness, it's driving new customer adoption on snowflake because people are insisting on doing data sharing through snowflake and you really see that happening in the financial services industry, which by the way shouldn't surprise you because the financial services industry has been sharing data for years and years and years.
除了仅仅保持用户黏性,这也在驱动新客户通过Snowflake采用数据共享,因为人们坚持通过Snowflake进行数据共享,你可以在金融服务行业中真正看到这种情况发生,顺便说一下,这不应该让你感到惊讶,因为金融服务行业已经分享数据很多年了。
Unfortunately, the data you've been sharing has been through FTP downloads, which is such an old technology or PDFs. And we can avoid all that. There's no reason why the whole concept of a bank statement going to a company is irrelevant. You can do data sharing so you don't have to actually transfer any data and you can just run your reconciliation directly against that in snowflake again.
很遗憾,您一直在分享的数据是经过FTP下载或者PDF格式的,这些都是旧技术。但是我们可以避免这些问题。银行对账单交付给公司的整个概念并不过时。您可以通过数据共享来完成,这样就不必实际传输任何数据,您可以直接在Snowflake中进行对账。
We're working on things like that for our own use case internally and it's much, much more efficient way of doing things. And more importantly, because the data isn't getting transferred, it's secure in government and you know exactly who's accessing it. Right.
我们正在为自己的内部使用案例开发这样的东西,这是一种更加高效的做事方式。更重要的是,由于数据没有被传输,所以这些数据是在政府部门中安全的,而且你可以确切地知道谁正在访问它。是的。
And just to continue down this thread a little bit, you talk about it in terms of creating stickiness from an investor perspective. I think one of the sort of holy grail that we're always looking for in our investments is where are there really defensible modes around companies because technology and software evolve so quickly.
继续沿着这个方向,从投资者的角度来谈谈,你谈到了创造粘性这个术语。我认为,我们在投资时一直在寻找的一个圣杯是哪些公司真正有可靠的防御模式,因为技术和软件发展得非常快。
It's hard to get a technology mode that remains durable, but ecosystems that people create around certain technologies and data sharing being one of them could potentially be that defensible mode. You talked about financial services. It could be a segue a little bit into sort of the industry focus because I'm sure this is probably one of the kernels of why you have this industry focus is to try to create these ecosystems.
获取一个持久的技术模式很困难,但人们创建的生态系统,包括数据共享,可能成为一种具有防御性的模式。你谈到了金融服务。这可能是过渡到行业焦点的一个导向点,因为我相信这可能是你拥有这种行业焦点的核心原因之一,是要试图创建这些生态系统。
Financial services is one. Can you walk us through some of the other verticals that you think you could develop these types of ecosystems in? Well, it's happening in the media streaming area with advertisers and media companies and data clean rooms is another form of data sharing and especially with all the privacy concerns today. That's definitely a key one.
金融服务是其中之一。您能向我们介绍一些其他垂直市场,您认为也可以在这些领域开发这种生态系统吗?嗯,在媒体流媒体领域,广告商和媒体公司正在实现这一点,数据“清洁房间”是另一种数据共享形式,尤其是在当今所有隐私问题的情况下。那绝对是关键之一。
Healthcare, there's all kinds of opportunities on both the payer side and as well as in pharma with the development of new drugs and stuff. There's a lot of data sharing that happens between companies in that. Many times the pharma companies use third parties to do part of the work on those things and that's an important piece as well too. You can pretty much apply it to any industry data sharing.
在医疗保健领域,无论是医保方面还是制药业方面,都存在着各种机会,尤其是随着新药的研制等。在这过程中,公司之间会进行大量数据共享,很多时候,制药公司会选用第三方机构来完成其中的一部分工作,这也是非常重要的一环。实际上,数据共享这个概念几乎可以应用到任何一个行业中。
It's funny when you talk to people. I was actually talking to someone the other day as a CIO of a bank and I was talking about data sharing and he's like, well, we don't really do any data sharing. I'm like, okay. That's what most people say. Then when you dig into it, oh yeah, we send these reports to fidelity. We get these things. You are doing data sharing. You're just doing it in an inefficient way. On the industries we even heard state governments. Yes. You imagine how many agencies are there and they all would like or would benefit from collaborating. So I think it permeates every industry.
和人交谈时很有趣。我前几天跟某家银行的CIO谈到数据共享,他说,嗯,我们没有做任何数据共享。我说,好的,这是大多数人说的话。但是当你深入挖掘,你会发现他们其实存在着一些数据共享的行为。只不过他们的方式效率低下。这种现象甚至存在于政府行业当中。你可以想象有多少机构想要或者受益于合作。所以我认为这种现象渗透到了每个行业。
And it all comes back to asking more questions of the data and utilizing the data more fully. If we go one step further and talk about the concept of data cloud that you guys talked to and now becoming a platform for application development, that's probably even a bigger expansion. It's kind of the market opportunity in terms of app dev.
一切都可以追溯到更多地询问数据并更充分地利用数据。如果我们再进一步谈论“数据云”这个概念,它现在正在成为应用开发的平台,这可能是一个更大的扩展。这实际上是应用开发市场的机会。
Why is Snowflake? Why is Snowflake the platform for doing this application development? And can you talk about some of the tools that there's a capability you brought on board like the native application framework and the stream lid acquisition that enable that application side of the data cloud to really come to fruition? Yes.
为什么选择Snowflake?为什么Snowflake是开发此应用程序的平台?您能谈谈其中一些功能吗,例如本地应用程序框架和流雷达采集工具,使得数据云的应用程序方面得以实现?是的。
为什么选择Snowflake作为平台往往是由于其众多优点,如可扩展性、易于管理和高效性。通过使用Snowflake,用户可以轻松地处理大量数据,而不需要花费大量时间进行准备和管理。
Snowflake还具有很多功能,如本地应用程序框架和流雷达采集工具,这些工具使得数据云的应用程序方面得以实现。本地应用程序框架允许用户在Snowflake环境中构建和运行应用程序,而流雷达采集工具允许用户轻松地获取实时数据流并对其进行分析。这些工具有效地支持应用程序开发,使得应用程序可以更加完善和高效地运行。
So the core thesis for us in this topic of collaboration is that in your organization that leverages second party data, third party data, second party and third party services will do better. And now at this point there are many studies where they show you will outperform your peers. If you've forgotten how to not only leverage your own data but how do you enrich and put your data in context.
因此,在合作主题中,我们的核心论点是,在利用二方数据和三方数据的组织中,利用二方和三方服务将会更好。现在,有很多研究表明,这样可以超越竞争对手。如果您忘记了如何不仅利用自己的数据,而且如何丰富并将自己的数据置于上下文中,那么现在是时候去找回它们了。
That's the concept of the data cloud for us and that is what is unique about Snowflake. Technology, yes, we can deliver technology and we're very proud of the technology we have. But when a customer buys into Snowflake, he buys into this data cloud and data cloud is where all these ecosystem of players, data providers is one form of partnership.
对我们来说,这就是数据云的概念,这也是Snowflake独特的地方。我们可以提供技术,我们对自己所拥有的技术非常自豪。但当客户购买Snowflake时,他也进入了这个数据云,而数据云是所有生态系统中的参与者、数据供应商等伙伴关系的一种形式。
But more interestingly, there's a lot of interesting IP, interesting business logic that organizations are creating and what we're doing with this concept of application platform and native apps in Snowflake is can you package that logic? Can you make it available to other customers? So now when a customer buys into Snowflake, they're buying through this ecosystem and we've seen customers that have passed on Snowflake. Like I'm not interested when they see some of the applications that are coming onto Snowflake they can do this data sharing that I can repurpose a team of 30 people that we're doing pipelines and ingestion and encryption and decryption. All of that goes away. That is the appeal and that's how we think of the data cloud unique for Snowflake.
更有趣的是,许多组织正在创造有趣的IP和商业逻辑,而我们在Snowflake中使用应用程序平台和本地应用程序这一概念,可以将这些逻辑打包成应用程序,并向其他客户提供。因此,现在当客户购买Snowflake时,他们是通过这个生态系统进行购买的。我们已经看到有些客户在看到一些正在进入Snowflake的应用程序后放弃了Snowflake。他们可以进行数据共享,我可以重新配置30人的团队,他们负责管道和数据传递等操作。这样做可以大大减少工作量,这也是数据云对Snowflake的独特吸引力所在。
No, I agree. And from a monetization standpoint, it all comes back to more questions being asked of the data and that's one of the really interesting things about Snowflake is such a straightforward pricing model, such a straightforward monetization model. It's actually a beautiful model that we really have one product, three different flavors of that product depending on which edition you want. But every new feature we have, our salespeople don't need to go in and get a PO out of a customer. They just need to go in and educate the customer so a customer can consume more and then the follow on capacity purchase orders follow.
不,我同意。从商业化的角度来看,这一切都归结于数据所提出的更多问题,这是Snowflake非常有趣的一面之一,它具有非常简单的定价模式和商业化模式。事实上,我们只有一个产品,有三种不同的版本,取决于你想要哪一个版本。但是,每个新功能的推出,我们的销售人员不需要向客户索取采购订单,只需要去向客户提供教育,以便让客户消费更多,然后跟随的容量购买订单就会随之而来。
The very simple model and I love it and the customer also because of our model, the way that we price, we sell a customer credit. A credit is a unit of measurement with the amount of compute you use and we charge you by the terabyte or terabyte of storage. You have and the beautiful thing of every software improvement, every hardware improvement that improves the price performance, you can do more with that same credit every year. So we become cheaper to our customers every year. And that's good because the better the price performance, the more workloads they move to us. The more performance, the speed at which we have. More workloads can come on to us that otherwise we weren't fast enough for. So our whole product roadmap is focused on more features that are going to drive consumption but then improving that price performance, the speed at which we operate.
这个非常简单的模型深受我和客户的喜爱,因为我们的定价方式是按客户所用的计算量出售信用点。信用点是一种度量单位,用于计算客户使用的计算量,并按照每个疊典字节或存储等同于一个疊字节的计量单位收费。每一次软件和硬件的改进都带来了更好的性价比,客户可以用同样的信用点完成更多工作。因此,我们每年都会让客户感到更便宜。这是很好的,因为价格性价比越好,客户就能把更多的工作负载交给我们。我们的整个产品路线图都专注于推出更多特性来推动消费,但同时也努力提高运行速度的性能和效益。
And we have a data. One thing to say, the other thing is we can show that the amount of compute credit that we generate per query, per question asked, it keeps going steadily down with public this year. The last three years, roughly 20% better economics for stove because of platform. And our customers see that. They don't even give them past your answers but better economics. And you can see that too. I think we're right about three billion queries a day running through snowflake. We cross three billion. I know as of last week we're eight million queries short of three billion a day. But you can see how the number of queries have grown in snowflake. The revenue doesn't grow as much why because the price performance improves the customers.
我们有一个数据。首先要说的是,我们可以展示出我们每次查询、每次提问所产生的计算信用的数量,在今年持续稳步下降。在过去三年中,由于平台的效益,炉子的经济条件大概好了约20%。我们的客户也看到了这一点。他们不仅得到了更好的经济效益,而且还能得到更好的答案。你也可以看到这一点。我想我们每天在雪花上运行大约30亿个查询。我们跨过了30亿的大关。我知道截至上周,我们还差800万个查询才能达到每天30亿。但你可以看到在雪花上查询的数量如何增长。收入没有增长那么多,为什么?因为我们提高了客户的价格性能。
We've got to improve price performance. We talked about a $248 billion tam but there's the address ability to that tam and you need to have the right price performance to address the entire tam as you improve that more and more of that potential market opportunity. You can serve as a more. Yes. We talk about the ML and AI opportunity within snowflake. And I think it's been a somewhat of an investor debate of whether a data warehouse, whether the snowflake architecture is correct for building ML, AI type of models on top of and workloads on top of. You guys announced a snow park for Python which I think makes it more applicable but Q20s was why the data warehouse and why it was snowflake is the right platform for building out these applications.
我们必须提高价格性能。我们谈论了一个2,480亿美元的市场,但是有针对该市场的可达性,并且你需要有正确的价格性能来覆盖整个市场。随着你不断改善这方面的表现,你可以为更多的潜在市场机会提供服务。是的,我们谈论了Snowflake中的ML和AI机遇。我认为这在一定程度上是投资者们的讨论,即数据仓库、雪花架构是否适合构建ML、AI模型和工作负载。你们发布了一个Python的Snow Park,我认为这使它更适用,但Q20的关键是为什么数据仓库及雪花是构建这些应用的正确平台。
Core to what we want to do and enable for our customers is deliver programmability of data. So how do I get value, how do I extract value out of my data without fading off governance and security. And that's what's different from what you will hear from everyone. Everyone has Python. We get as a love. Why did it take you two or three years to incorporate Python into snowflake? Because incorporating Python in an unsecured way is easy. We can do it in a couple of weekends. But then you can ask CIOs, how do you know that your data science team did not download some library from the internet and it may have had a vulnerability and potentially exfiltrated data. And that's where the answers get a little bit less clear.
我们想要为客户实现的核心是数据可编程性。那么如何从数据中获得价值,如何在不降低治理和安全性的情况下提取价值。这与你从其他人那里听到的不同。每个人都有Python。我们非常喜欢它。为什么你要花两三年时间将Python集成到Snowflake中?这是因为以不安全的方式集成Python很容易。我们可以在几个周末内完成。但是你可以问CIOs,你如何知道你的数据科学团队没有从互联网下载某个库,并可能存在漏洞并潜在地外泄数据。这就是答案变得不太清晰的地方。
The networking team was in charge. What we offer and I'll get to your AI part of the question. What we offer is a secure way to program data. And when we say program data it can be just transform data or it could be doing AI and ML. So for us, AI ML is one additional workload that we want to support running close to the data in a secure fashion.
网络团队负责。我们提供的是一种安全的数据编程方式。当我们说编程数据时,它可以只是转换数据,也可以是进行人工智能和机器学习。因此,对于我们来说,AI和ML是我们想要支持在安全的方式下紧密运行数据的额外工作负载。
And then you can say you want to do training. We have customers coming into something to do training. You want to do machine learning scoring. We have customers coming into the scoring. And at our user conference we introduce this low latency storage mode we call unistore, which is very low, very fast reads and writes. That's very common for online feature stores, online recommendations, applications of ML.
然后,你可以说你想要做培训。我们有顾客来做培训。你想做机器学习的评分。我们有顾客来评分。在我们的用户会议上,我们介绍了这种低延迟存储模式,我们称之为 "unistore"。它的读写速度非常快,非常适用于在线特征存储、在线推荐和机器学习的应用。
Then you come and say, well there's a new thing called language models. Language models is nothing but here's another form of pre-trained machine learning. I want to be able to score that based on data that I have in Snowflick. I may want to be able to fine tune that based on data having Snowflick. And for us, it's a continuum. I'm not trying to dismiss the importance of AI but what is really important is do everything you want to do. Program data, do AI, do proprietary computations, do so without trading off security, governance, policy, privacy.
然后你会说,现在有一种新的东西叫做语言模型。语言模型只是另一种形式的预训练机器学习。我想根据我在Snowflick中拥有的数据来评分它。我可能想要根据在Snowflick上的数据进行微调。对我们来说,这是一个连续的过程。我并不是试图否认AI的重要性,但真正重要的是能够做任何你想做的事情。编程数据,做AI,进行专有计算,而无需牺牲安全、治理、政策、隐私。
That's the value problem of Snowflick and it resonates with customers. And it's something I hear a lot when I'm talking to CIOs, particularly in regulated industries. When they're thinking about these large language models and stuff like chat, the security implications haven't really been explored. It is a real threat of data leakage on a go-forward basis. But if I'm hearing you correctly, today have companies that are utilizing Snowflick for training these models? Yes. And for sure, we have no only customers leveraging Snowflick for machine learning. Part of it, there is Snowflick for Python integration. Enable that.
这是Snowflick的价值问题,它与客户共鸣。当我和首席信息官(CIO)交谈时,尤其是在受监管行业中,我经常听到这样的问题。当他们考虑使用这些大型语言模型以及类似聊天的东西时,安全问题并没有得到真正的探讨。这是真正的数据泄漏威胁。但如果我听到你的话正确,今天有公司利用Snowflick来训练这些模型吗?是的。当然,我们不仅有客户利用Snowflick进行机器学习,还有针对Python集成的Snowflick。
We introduce a type of cluster that has more resources. We call it Snowflick warehouses, which are just about this. The one piece that you can say, well, you don't have is GPU support. That is for cases that's where this deep learning. And you can stay tuned. We'll be sharing more about this at our user conference in June. But fundamentally, we just think about it. The broad vision and broad goal for Snowflick is bring computation. Whatever the nature it may be, to run closer to the data, and AI and ML is just one such example.
我们推出一种拥有更多资源的集群类型,我们称之为“雪花仓库”,它就是这个意思。唯一可以说我们没有的就是GPU支持,这是针对深度学习情况的。请继续关注,我们将在6月的用户大会上分享更多关于此的信息。但是,基本上,我们只是考虑它。雪花的广阔愿景和目标是将计算带到更接近数据的地方,而AI和ML只是其中的一个例子。
Got it. Perfect. I want to dig a little bit into a unistore. That's something that you guys talked to us a lot about at the last annual stage. And it enables Snowflick to now address more transactional workloads. And for the broader audience, there's analytical workloads and transactional workloads. And historically, never the two shall meet.
明白了,太好了。我想深入了解一下unistore。这是你们在“最近年度舞台”上经常和我们谈论的东西。它使得Snowflick能够处理更多的交易工作负载。对于广泛的受众来说,有分析工作负载和事务工作负载。历史上,这两者从来没有交集。
Today, given sort of the computation resources, you guys have at hand, and it's not constrained, it's now more amenable. You can bring those two together. So one, you talked to us about the underlying technology that enables you to bring those two together. And two, what's the market opportunity that opens up when you can look at the data from both perspectives, both in terms of using it for transactions that also for the deep analytics?
今天,考虑到你们手头拥有的这种计算资源并没有受到限制,现在更易于将它们结合起来。因此,你们可以将这两者结合起来。一方面,你们需要向我们介绍使它们结合起来的底层技术。另一方面,当你们能够从两个方面观察数据时,利用它们进行交易和深度分析也会带来什么市场机会呢?
Yeah. So I'll rewind a little bit on database history. In the very early days, a database was a database was a database and it did both transactional analytics. It's only that before my time. Way before my time, or I got the tail end of that. And then specialization happened.
是的。所以我会稍微回顾一下数据库的历史。在早期,数据库只是一个数据库,可以进行事务和分析。那是在我出生之前,我只能了解到那个时代的最后一部分。之后,专业化逐渐发生了变化。
And for example, Terra data, credit what credit do, say we're going to build a database focused on analytics. And many others follow the verticals, the thesis, et cetera, and Oracle and the B2 and others went on the transactional site. And for 20, 30 years, they were going to separate tracks. And as you said, they would never combine because the specialization was for each type of use case.
例如,以Terra data为例,它为数据分析建立了一个数据库,信用有信用的说法。其他公司也会根据垂直领域、论文等方面进行跟随,而Oracle、B2和其他公司则专注于事务处理方面。在过去20、30年中,它们走上了不同的轨道,因为它们的专业化适用于每种使用情况。正如您所说,它们永远不会结合,因为专业化是为每种用例而设计的。
What's changed and what's different, which is the question we get asked on the book, okay, if this thing has never happened, why do you think you have a shot at succeeding years? The cloud helps us present a unified product, a unified experience for our customers. Even if behind the scenes, there are different ways to store the data. And that's what Unistore does. The implementation of Unistore, we call it a hybrid tables, hybrid because they have a storage system optimized for analytics and storage system optimized for fast, region rights.
书中经常被问到的问题是有什么变化和不同之处,如果这件事从未发生过,为什么你认为你有机会在未来几年成功?云帮助我们为客户呈现出统一的产品和体验。即使在幕后,存储数据的方式是不同的。这就是Unistore的作用。我们实现了Unistore,并称其为混合表,之所以称之为混合表,是因为它们具有针对分析优化的存储系统和针对快速区域写入优化的存储系统。
But we can behind the cloud, tie all the details and data replicated, that have moved back. And what this does for us is now we enable customers to store data in Snowflake, build applications, application state or machine learning pipelines or machine learning inference and low latency. We're very fast reads, very fast writes, but also that they let seamlessly available for analytics. So it's the technology and the cloud, the fact that we're delivering a hosted service that enables us to do this. And I believe it's a big part of our application stack.
我们可以在云端背后,把所有的细节和复制的数据捆绑起来,然后迁移回原处。这样做的好处是,我们现在可以让客户把数据存储在Snowflake中,构建应用程序、应用程序状态,或者机器学习流水线或机器学习推理和低延迟。我们读取速度非常快,写入速度也非常快,而且可以无缝地为分析服务。这是技术和云端的结合,我们提供托管服务使我们能够做到这一点。我相信这是我们应用程序堆栈的一个重要组成部分。
But if you want to say something about the market opportunity, yeah, no, it's, well, we don't know how big the market. It's really a good opportunity, but I think it's important to, I think it will be a revenue. First of all, the product is still a private preview today, it's on public preview. It will be in public preview at the end of this year. We've learned a lot from customers and we're revising some of the stuff on the engineering side.
但是如果你想谈论市场机会,我们其实不知道市场规模有多大。虽然这是一个很好的机会,但我认为重要的是,它会产生收益。首先,这个产品目前仍处于私人预览阶段,还没有公开发布。它将在今年年底进入公开预览阶段。我们从客户那里学到了很多,并在工程方面进行了一些修改。
But that will have a impact on margins because of the fact that there's du- there's duplication of data. Definitely it will drive revenue, but it will, it's not going to cause our margins to decrease, but it puts a gate as to how big the margins can get, the product margins in the company. But definitely opens up a massive market opportunity for us as well too, to be determined how big that is.
但是这将会对利润率产生影响,因为数据存在重复。这肯定会推动收入增长,但它不会导致我们的利润率下降,而是限制了公司产品利润率的扩大。但是,这肯定也为我们打开了一个巨大的市场机会,只是这个机会有多大还待确定。
Yeah, and I would add that the bigger goal for us is enable full applications to run inside Snowflake. And if you look at the elements of an application, there's the core storage, so we have Snowflake Analytics as well as Unistore. You want a metal tier to be able to do processing that's where Snowpark fits in, and you want a presentation tier which is where Streamlit fits in, right? The combination of all of those, change the art of what's possible and how we think modern applications will be built and deployed in a secure way.
是的,我想补充一点,我们的更大目标是使完整的应用程序能够在Snowflake中运行。如果您观察应用程序的组成部分,有核心存储,因此我们有Snowflake Analytics和Unistore。您需要一个金属级别来进行处理,这就是Snowpark的作用,而您需要一个演示层,这就是Streamlit的作用,对吗?所有这些的结合,改变了可能性的艺术,以及我们所思考的现代应用程序的构建和安全部署方式。
Got it. That's a good summary. I want to shift gears a little bit and talk about the business model and get into near-term results and maybe just stick with the theme of history lessons. I think one of the really interesting things about Snowflake is like how pure of a consumption model it is.
明白了,这是一个很好的总结。我想换个角度,谈谈商业模式,重点可能在于近期成果,坚持历史教训主题。我认为Snowflake非常有趣的一点在于它有多么纯粹的消费模型。
And if we think about it holistically from where we came from with perpetual license models where all the risk was put onto the end customer, like you got to figure out how to set it up, you have to figure out how to get productivity out of it, but upfront you give us a couple million dollars. With Snowflake, nobody's paying you until they're starting to run queries against the day.
从我们一直使用永久授权模型开始思考,这种模型把所有的风险都转嫁给最终客户,比如你必须自己想办法搭建系统,必须自己想办法提高生产力,但你需要提前付给我们几百万美元。然而,对于 Snowflake,除非客户开始查询数据,否则没有人会付款。
Well, correct that. They're paying us many times up front but they're not incurring the expense. They're not incurring the expense, right? There's a commitment, but you're taking on a lot more of the risk. Yeah, we take on the risk, and that's why it's super important that we are there for our customer success and why we spend a lot of time and why we insist our salespeople stay engaged with customers in the seat model, and I know this one, I was the CFO of ServiceNow, and I know we buy a lot of licenses from other people.
“好的,纠正一下。他们事先付了我们很多倍的费用,但他们并没有承担费用。他们没有承担费用,对吧?虽然有承诺,但你们承担了更多的风险。是的,我们承担风险,这就是为什么我们非常重视与客户的成功合作,并且花费大量时间,坚持我们的销售人员与客户在座席模式下保持联系的原因。我知道这一点,因为我曾经是ServiceNow的首席财务官,我知道我们从其他公司购买了很多许可证。”
It's painful when you buy a license and you start having the expense even though you may not start using it for six months. And so yes, we do bear that at Snowflake, but the benefit of that though is just as you can see a slow down, if people are tightening their belts, you can see an acceleration in our businesses will too, and people have more visibility into their business.
当你购买了许可证,即使你可能在六个月内没有开始使用它,你开始承担费用是非常痛苦的。所以,是的,我们在Snowflake承担了这个费用,但好处也同样显而易见,就像你可以看到当人们勒紧裤腰带时,我们的业务也会加速发展,人们对他们的业务有更多的可见性。
So people taking advantage of that flexibility during a tightening spending environment, and just to bring it back to the current results and what we've seen throughout 2022, obviously customers are taking advantage of that, and we've seen optimization in all sorts of cloud models including Snowflakes.
在紧缩的支出环境中,人们利用这种灵活性,而且回到当前的结果和我们在整个2022年中所看到的,显然客户正在利用这一点,在各种云模型中包括Snowflakes中看到了优化。
How do you get an assessment of where we are in that cycle? Yeah, so I think optimization is an overused term by many companies today. We've been talking about optimizations as far as two years ago, and at our financial analyst day we talked about this is this is nothing unique and this will be ongoing with any customer, but there are no big optimizations out there.
你如何评估我们在这个周期中的位置?是的,我认为很多公司今天过分使用了优化这个词。我们两年前就开始谈论优化,而在我们的财务分析师日活动中,我们谈到这并不是独特的,这会随着任何客户的进行而不断进行,但不存在大规模的优化。
And optimizations just to be clear what they are, is we find instances where snow people have not written the most efficient queries that are taking up too much compute. We spend time on the professional services time to help them rewrite, re-engineer the query so they use less compute, but one of the biggest and low hanging fruits on optimizations, we've seen customers store data that they've never accessed, and why are you doing that? We've seen customers store the data twice in Snowflake when you don't need to.
为了更清楚地表达,优化的意思是,我们发现有些Snowflake的使用者编写了效率不高、占用计算资源过多的查询语句。我们会花费专业服务的时间帮助他们重写和重构查询,以使其使用更少的计算资源。但是,优化中最大、最容易实现的收益是,我们发现很多客户存储了从未访问过的数据,这样为什么要这样做呢?我们也发现一些客户在Snowflake存储了冗余的数据,这实际上是不必要的。
We've seen customers where they would choose bigger warehouses than what they really need. They would disable the auto suspend function. Well, today we help you pick the right size warehouse that you need and take away a lot of that. You still customers can still choose, but we help you size the warehouse correctly. When you disable the auto suspend function there's a lot more alerting that happens on that, and we're monitoring constantly to make sure that warehouses aren't left hanging. That's what optimizations are for us. And we will continue adding product capabilities to do all of this proactively or automatically for customers. Nobody wants to cycle up, I grew up, I optimize, I grew up too much. We believe in you're always optimized and that's good for everyone.
我们曾经遇到一些客户会选择比实际需求更大的仓库,他们会禁用自动暂停功能。今天,我们将帮助您选择合适尺寸的仓库,并解决很多问题。客户仍然有权选择,但我们将帮助您正确尺寸仓库。当您禁用自动暂停功能后,将出现更多警告,我们会不断监视以确保仓库不会被忽略。这就是我们进行优化的原因。我们会继续添加产品能力,为客户主动或自动完成所有这些操作。没有人想要这样的循环:起初优化,然后发现增长过于快。我们相信您总是优化的,这对每个人都有好处。
And I have a team of people that literally look at spikes in revenue on a daily basis, and when they see something, we reach out to the customer driven by finance with the rep to do that, understand what's going on. You may say, why am I doing that? I know if we have an unhappy customer that they left a warehouse running or they're using snowflake inefficiently, they're going to ask for credit back. And so I'd rather get in front of that. But more importantly, we reforcaster revenue on a daily basis based upon the prior days consumption. And if I'm incorrectly reforcasting on spikes that aren't real spike, like ongoing consumption, then I have a problem. So we do that. I don't know a single vendor that's ever reached out to me to tell me when I'm consuming too much of something. So you feel comfortable that you've flanned out that curve. If you look at that, you've taken out the any excess. There are no big optimizations that are unaware of. And it's now, it's a matter. There's not some small ones, but there's no big ones out there when I look at the top customers.
我有一个团队,每天都会关注收入的波动,当他们发现需要时,我们会与客户联系,由财务人员与客户代表一起了解情况。你可能会问,为什么我要这样做呢?因为我知道,如果我们有不满意的客户,他们可能会要求退款,因为他们发现仓库管理不当或使用效率低下。因此,我更愿意预先预防这种情况。但更重要的是,我们每天都会根据前一天的消费情况重新预测收入。如果我错误地预测了不是真正波动的波动,例如持续的消费,那么我就有问题了。所以我们这样做。我不知道有哪个供应商曾经联系过我,告诉我我消耗了太多的某种东西。因此,你可以放心,你已经平稳了曲线。如果你看一下那个曲线,你已经将任何多余的东西都去掉了。没有我意识不到的大优化,现在只是一些小的问题了。即使对于顶级客户,也没有大的问题。
Got it. So when we think about the adjustment of the 40 year kind of revenue guidance that you did on the last conference call, that was less about optimizations or not about optimizations about consumption patterns. It's about customers ramping more slowly. And what I would say is early adopters by their nature tend to move faster. And a lot of our early customers were, let's get up and running on Snowflake, who cares about costs and we'll worry about optimizing later the more. I don't want to say, um, laggards, but the later adopters tend to be more methodical. They tend to be more cost conscious. Those, a lot of our early customers were the digitally native companies that were very fast moving and were growing so quickly, they didn't really care about costs. But when you're dealing with well-established global 2000 companies, these guys have always cared about costs and they just move at their own pace. And what we're seeing is we've landed so many of these large customers over the last three, four years. They just grow slower than these digitally native early adopters. Got it. They're still going to get to the same end and state. Okay. So the destination remaining is the same. It just takes longer and longer to get there. Yeah, it gets there.
明白了。所以当我们考虑你在上次电话会议上做出的40年收入指导调整时,这与优化无关,而是与消费模式有关。这是因为客户的启动速度变慢了。我想说的是,早期的采用者本质上往往移动得更快。我们很多早期客户的想法是,让我们开始使用Snowflake,管它什么成本,等到后面再考虑优化。我不想说是“落后者”,但后来的采用者往往更加谨慎。他们更注重成本。很多早期客户都是数字原生公司,非常快速并且增长迅速,他们并不十分在意成本。但是当你与已经成立的世界500强公司打交道时,这些公司一直非常注重成本,并且只按自己的节奏前进。我们看到的是,在过去的三四年里,我们与如此多的大客户合作,但相对于这些数字原生的早期采用者,他们的增长速度变慢了。明白了。他们最终还是会达到同样的目标和状态。好的,只是需要更长的时间才行。是的,最终会到达。
And maybe one of the dynamics has been really interesting in the model throughout that the year is the large customer growth has still been very robust. That in the last three quarters of each been the largest net new additions into the million dollar plus spenders. Could you take in with us a little bit about the sort of the life cycle of that million dollar plus customer? How long did it take them to get there? I think the average million dollar plus customer now is like 3.7, 3.7, 3.7. Three point seven. Like, what's the long as it take them from when they cross a million to get to sort of like that average? So, you know, when we sign up a new customer, a global 2000 is a little over 100 grand is what they start at. A normal non-global 2000s in the 50 to 60 thousand a year and they quickly grow it usually will take a global 2000 to get that million dollars two to three years to get there. Why? Because some get there much faster, but they generally move pretty slow these companies. Got it.
也许模型中非常有趣的一个因素是,大客户增长仍然非常强劲。在过去的三个季度中,每个季度新增的百万美元以上的消费者数量最多。能否跟我们谈谈这些百万美元以上客户的生命周期?他们从成为客户到达这个平均水平需要多长时间? 我认为,现在的百万美元以上客户平均需要3.7年,对吗? 那么,当我们签约一位新客户时,全球前2000家企业起步收入一般为超过100,000美元。一般非全球前2000家企业则一年的收入在50到60,000美元之间,然后通常会快速增长。全球前2000家企业要花费2到3年时间才能达到百万美元的水平。为什么?因为有些企业可能会更快地实现这个目标,但这些企业通常会慢慢成长。明白了。
Well, I want to shift gears to the margin side of the equation because that was the other really pretty spectacular part of the equation if you look back at calendar 2022. Consumption models aren't supposed to see expanding margins as growth flows down. It should be harder for you guys to grow margins. So, first of all models are mechanically geared that when growth flows down, you could just see more margins.
好的,我想把注意力转向方程的边际部分,因为如果你回顾2022年的日历,那也是另一个非常惊人的方程部分。消费模型不应该看到边际扩张作为增长下降的流出。你们应该更难提高边际。所以,首先,模型机械地调节,当增长下降时,你可能只能看到更多的边际。
But you guys have a really robust expansion in your overall free cash flow margins during 2022. How are you able to do that? And if the demand environment gets better and the consumption picks up, shouldn't that be incremental even sort of more positive from margins on and go forward business?
你们公司的整体自由现金流边际在2022年有很强的扩张,这是如何实现的?如果需求环境变得更好,消费增加,这不应该对边际和未来业务更有利吗?
So, we've been, I've been with the company enough for a little over three and a half years and since day one, I remember that first year when I joined, we were expected to burn 220 million and we'll quickly turn that around. We've always been focused on free cash flow and I would say it's revenue growth, product margins, and free cash flow. And you know, it's pretty simple math. As to how that's working, we continue to show product margin improvements. We continue to show operating margin improvements.
所以,我已经在这家公司工作了三年半,自从加入的那一天起,我记得第一年我们的预期支出是2.2亿美元,但我们很快就扭转了局面。我们一直关注自由现金流和收入增长、产品毛利率和自由现金流。你知道,这是相当简单的数学问题。就这样,我们继续展示产品毛利率的提高,也继续展示营业利润率的提高。
I will say what's kind of surprised me is I was expecting early on more of a shift in payment terms with our customers. Most of our customers still, they signed a three-year contract or one-year contract and passed annually in advance. 80% plus of our customers still do that. I have expected customers to want to move to quarterly or monthly payment plans. Why? Because the cloud vendors give everyone monthly payment terms and that is an option to customers. We give them that option, but it's all about discounting and they would rather get a higher discount and pay up front.
我想说的是,令我有点意外的是,我在早期就预计我们的客户在付款方面会更加翻新。大部分客户仍然签署三年或一年的合同,并提前一年支付费用。80%以上的客户仍然这样支付。我原本以为客户会想转向每季度或每月的付款计划。为什么呢?因为云服务供应商给每个人都提供了月付款方案,这对客户来说是一种选项。我们也给他们这个选项,但这取决于折扣,他们宁愿获得更高的折扣并一次性付款。
I do think with people earning real returns on cash balances overnight now that there will be a shift in that and that's one of the reasons why we kept our free cash flow flat at 25% next year. That's one piece but then also we got some surprise early payments in January that I wasn't expecting that influence that made that free cash flow higher in Q4 than it otherwise would have been.
我认为随着人们现在在现金余额上获得实际回报,会有一个转变,这是我们明年将我们的自由现金流保持在25%的原因之一。这是一个方面,但我们在一月份得到了一些意外的早期付款,这是我没有预料到的,它影响了Q4的自由现金流比它本来应该的更高。
One other thing I want to make sure we touch on we have about a minute left is the expansion of the AWS relationship. AWS, obviously a major infrastructure partner for you guys as well, but you've well expanded that relationship. It's not just being in the market place. There's go to market commitments being made on both sides of the equation.
还有一个我想确保我们谈及的事情,我们只剩下大约一分钟的时间,那就是AWS合作关系的扩展。AWS显然也是你们的重要基础设施合作伙伴,但你们已经扩展了这种合作关系。不仅仅是在市场上存在,两个方面都做出了营销承诺。
You dig in a little bit about that of what you and Amazon are now doing together on on and go to market. Sure. We have committed head count out of AWS aligned to our verticals globally as well. We're committing head count to Matt, we've had those head count anyways, but we're matching our head count as well too on the on the alliance side. There's more dollars that are committed for migration funds to a snowflake on AWS.
你可以进一步了解一下你们和亚马逊正在合作的有关市场推广方面的情况。我们在全球范围内承诺了与 AWS 相关的人力资源来支持我们的垂直业务。我们已经承诺了人力资源来支持 Matt,不过我们也会匹配我们在联盟方面的人力资源。此外,我们还拨出更多的资金来支持在 AWS 上进行数据迁移。
There's a lot more free credits. There's a lot of POCs that we run when those POCs could be a new customer, but then also when we're looking at doing snow park, we offer free credits to customers to do e-vails. That stuff is funded by the cloud guys. We fund some of it and they're willing to make the throw money in. It's a pretty big financial commitment. We're making a big financial commitment to them. They're making a big financial commitment to us as well too.
还有更多的免费信用。我们会进行很多 POC(概念验证)实验,这些实验可能会成为我们的新客户,当我们考虑进行雪场项目时,我们会向客户提供免费信用来进行网上交易。这些活动是由云服务公司资助的。我们部分资金支持这些活动,他们也愿意投入资金。这是一个相当大的财务承诺。我们对他们有一个大的财务承诺,他们也对我们有一个大的财务承诺。
It also promotes AWS is really good about everyone thinks you compete with AWS Redshift. They're going to they talk about all these product improvements. The reality is, in large accounts, AWS partners with us out of the gate because they want to see those customers land in AWS. History has shown that snowflake helps those customers land in AWS. That's good for AWS because they can sell a lot of other software services around snowflake.
它还宣传了AWS真的是很好,大家都认为你们与AWS Redshift竞争。他们会谈论所有这些产品改进。实际情况是,在大型客户账户中,AWS与我们一开始就合作,因为他们希望看到这些客户落户AWS。历史表明,雪花帮助这些客户落户AWS。这对于AWS来说是很好的,因为他们可以在雪花周围销售许多其他软件服务。
That's standing. Unfortunately that takes us to end of our lot of times a lot, but Mike Christian, thank you so much for joining us today. Thanks for having us. Bye.
那就是今天的节目。不过我们的时间也已经到了很多次的尽头,但Mike Christian,非常感谢您今天的加入。谢谢您的参与。再见。